Webinar 041
Application of graph neural networks to predict explosion-induced transient pressure and flow fields
FABIG Members: Log-in to access all FABIG resources LOG IN
Speaker(s): Ginevra Covoni
Organisation(s): Imperial College London
Duration: 62 min
SUMMARY
Explosions are complex phenomena involving rapid gas expansion, increased pressure, and elevated temperature. Accurate modelling of explosions is crucial for compliance with safety regulations. Some applications require modelling of large geometric domains with high resolution in both time and space. The often prohibitive computational cost of such modelling drives designers towards simplified procedures and approximate numerical schemes, ultimately increasing costs and jeopardizing safety. Machine learning techniques can be applied to address this problem.
This presentation illustrates an application of graph neural networks (GNNs) to simulations of the pressure and flow fields following a sudden explosion. A training dataset was constructed conducting URANS simulations of impulsive events in OpenFOAM. A cubic volume of air enclosed in rigid walls was modelled, which also encompasses one or more rigid obstacles of random shape, position and orientation. At time 0, one or more subsets of the cubic volume are initialized to have a higher pressure than the rest of the domain. The ensuing shock initiates the propagation of pressure waves and their reflection and diffraction at the obstacles and walls, creating complex transient flow conditions. The time histories of the fields of pressure, temperature and velocity obtained from a set of ~60,000 realisations of such simulations were recorded to serve as a training database.
A GNN algorithm recently proposed by Deepmind (MeshGraphNets) was modified and adapted to this problem. The model implements an Encode-Process-Decode structure, enabling message passing between neighbouring nodes, which can directly operate on arbitrary meshes, as is illustrated in the talk. During the training, the model learns the evolution of thermodynamic quantities in time and space as well as the effect of the boundary conditions. After training, the model can very quickly compute such evolution for unseen geometries and arbitrary initial and boundary conditions, exhibiting good generalization capabilities. The accuracy and efficiency of the predictions is demonstrated in the talk, followed by plans for further developments.
Online purchase options:
Non-members are able to purchase access to online streaming of the online lectures and webinars.
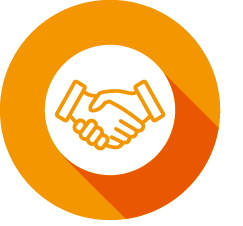